A Quick Guide on Media Effectiveness Measurement
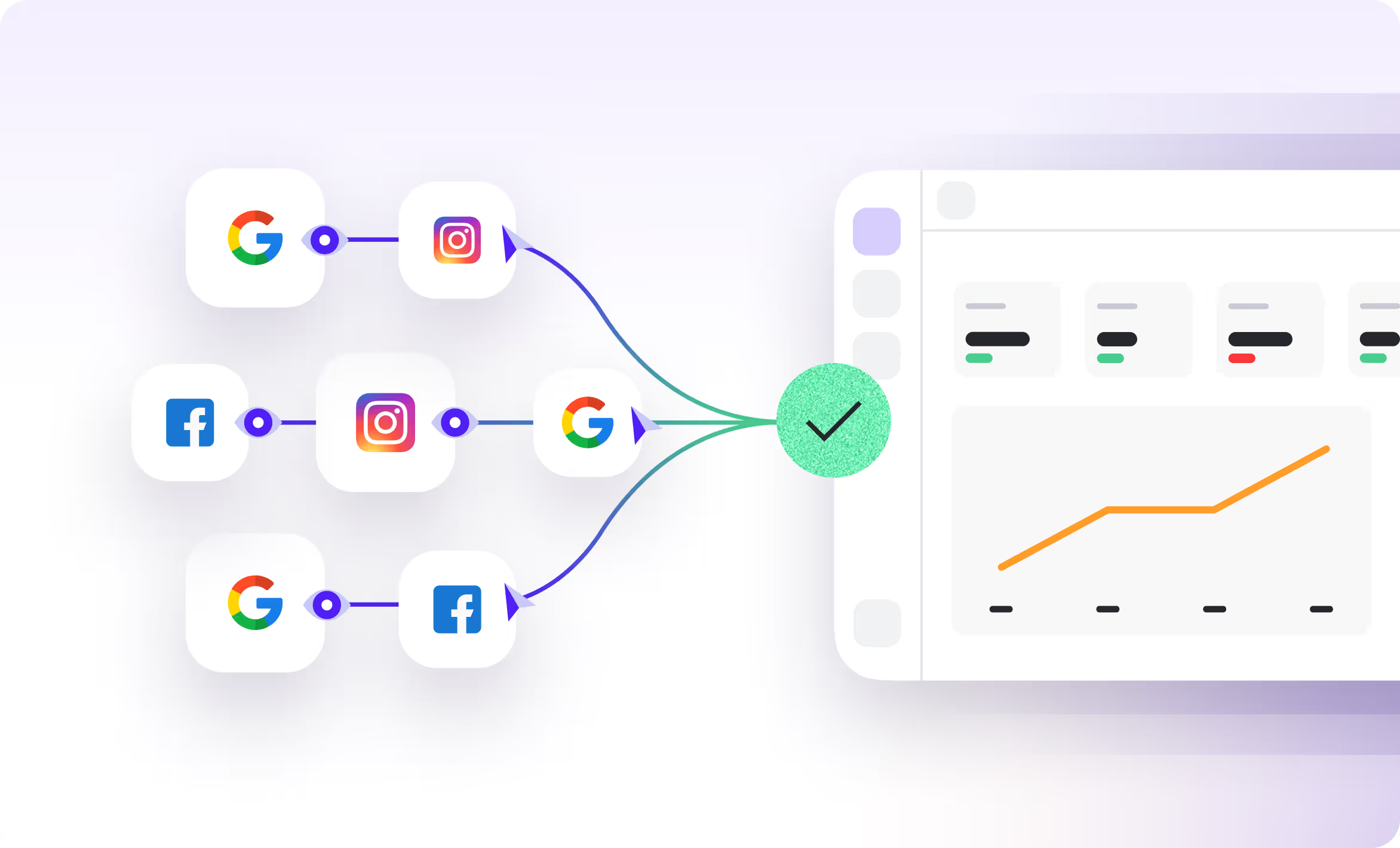
Media effectiveness measurement has significantly evolved in recent years, especially in digital marketing. The perceived 'doom' brought about by the depreciation of third-party cookies and the requirement for consent to track has shifted the focus toward privacy-centric solutions. As standards have risen, marketers are challenged to find new alternatives to bridge the data gaps to answer two key questions:
- What is the full impact of my media investments across channels?
- How can I best optimize my media investments?
What you will learn
Introducing Media Effectiveness Measurement Tools
Media effectiveness supports business growth by answering two key questions:

Traditional attribution models (rule-based) such as last-click and Ad platform-specific attribution (the ones used in Google ads and Facebook Business manager) are now being questioned within organizations. Marketers who assess multiple marketing channels no longer rely solely on data from advertising platforms due to issues with duplicate data. When the revenue reported by all advertising platforms is summed, it often totals a figure five times higher than what is recorded in the shop system.
They also recognize that rule-based attribution models like last-click and first-click are only useful in specific situations, such as when there is significant traffic at the bottom of the funnel, and there is a need to identify which channels effectively convert this traffic at the last stage.
Other basic attribution models, such as position-based, U-shaped, or decay models, also fail in their quest to help marketers allocate the media budget to the best-performing channels. They evaluate the quality of the touchpoint based on its position and ignore more critical signals, such as user behavior on the website during the session or the distance between touchpoints. Essentially, they are not capable of determining whether a conversion would still happen without that specific touchpoint (incrementality).
In response to these challenges, data-driven attribution models have emerged. They aim to incorporate more calculations and variables, but with two main downsides: in most cases, they are a black box (no one explains how they work, making it difficult to understand incrementality — in the worst case, they are biased, like GA4 which favors Google own media); second downside they rely on clicks. This means all users who do not click on an ad but purchase later are not correctly attributed. Evidence of this issue is seen in the disproportionately high share of conversions in direct, branded and organic search in click-based attribution, regardless of the quality of tracking or model.
Other Media Effectiveness Measurement Tools
In response to the limitations of click-based measurement, two new tools have gained importance: Media Mix Modeling (MMM) and Incrementality experiments.
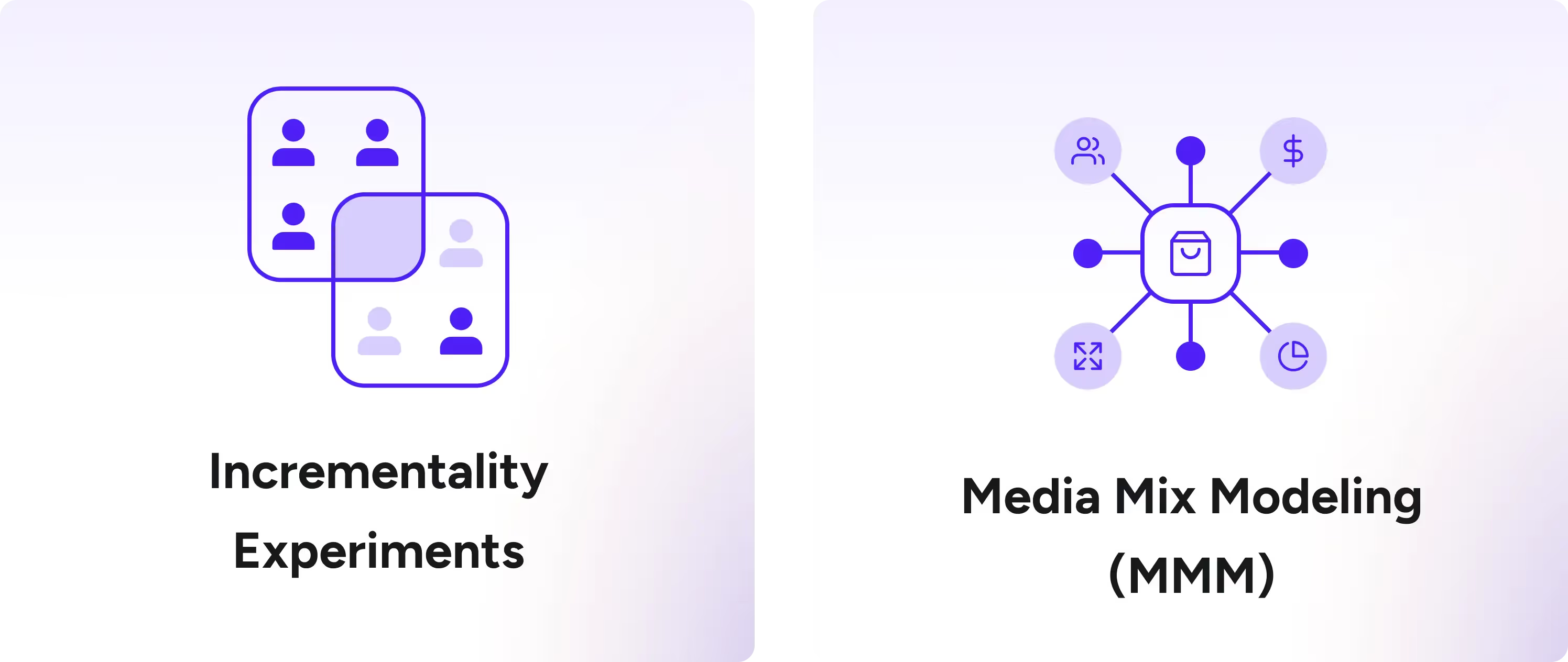
Incrementality Experiments
Incrementality experiments use randomized controlled experiments to compare changes in consumer behavior between groups that are exposed to or withheld from marketing activities, while keeping all other factors constant. Among all methods, they are the most rigorous in testing causality. Typically, these experiments are conducted quarterly and are best suited for providing a snapshot of a specific strategy at a particular point in time.
However, they are not ideal for daily monitoring and are difficult to scale. Calibration should be done continuously and across as many channels as possible to ensure representativeness.
Media Mix Modeling (MMM)
On the other hand, Media Mix Modeling (MMM) is a high-level approach that utilizes advanced statistics to understand what drives sales.
It measures the efficiency of media investements on top base sales. It also considers external factors that impact sales, such as seasonality, pricing, and economic conditions. MMM is conducted biannually and requires modeling based on at least two years of historical data, making it costly to implement.
Which Tool is the Best?
Actually, that's the wrong question. No single tool has all the answers. You will need a combined approach that uses each tool’s strengths to fill in the gaps. It really depends on the analytics maturity stage of your company and, of course, your budget.
Typically data-driven attribution models are used for daily channel and campaign tactics/optimizations. Media Mix Modeling (MMM) is ideal for capturing the long-term impact of brand activities in both digital and offline advertising, while incrementality tests excel in determining the causality of crucial strategies, especially when making significant investments in a new channel.
For example, advanced-stage companies develop an annual test and learning plan, where insights from each tool are used to continuously improve and complement each other. For example, results from incrementality experiments calibrate and validate MMM, and MMM can be used to complement attribution results.
AI Click & View Attribution
Used as complementary assets, marketing measurement tools will boost your performance. The internal capabilities of your team play the most important role. As the saying goes, you learn to walk before you run.

But…innovation needs to be at the core to combine these tools successfully. Your daily channel and campaign tactics deserve tools that represent reality as closely as possible. Therefore, Kickbite pushed the limits of an AI Click attribution model and developed the AI Click & View Attribution model. Tested and reiterated over 12 months with data from over 30 ecommerce shops. The impact of view conversions is now considered.
How Does it Work?
In a nutshell, you start with the results from the AI Click Attribution model and calibrate these based on statistical models that measure the strength of the relationship between view conversions and the baseline sales.

A Step-by-Step Guide
1. Extract Data.
Extract the impression and view conversion data from the advertising platforms at the ad level via the API.
2. Create Calibration Multiplier.
Create a calibration multiplier based on statistical models. This identifies the strength of the relationship between the view conversions and baseline sales (including direct, brand search, and organic search).
3. Calibrate Social Ads.
Calibrate the results form the AI Click model using the calibration multiplier.
4. Match Baseline with Revenue.
Adjust the baseline channels (direct,brand and organic search) to match it with your backend revenue.
5. Recalculate KPIs.
Recalculate all other KPIs like AOV, new orders and Return on Adspend.

Is AI Click & View Attribution the Perfect Solution?
We don't dare to say that. There is always room for improvement, and hybrid methodologies (calibration of Attribution through Statistical Modeling) have the following limitations:
1. No live data
The processing of data requires more time. In the case of the AI Click & View, there is a one-day delay. This means that today, you will see the data from yesterday.
2. Dependency on external data sources
For the AI Click & View, conversions from Ad platforms are required input variables.
3. Only channels only
Does not consider offline channels or conversions.
4. Incrementality tests
Best tool to establish causality.

5 Recommendations for Better Measurement
Evolving Measurement Techniques. Digital marketing measurement has evolved, you need to move from third-party cookies to privacy-centric tracking across all marketing channels.
Limitations of Traditional Models. Traditional models like last-click (and all rule-based) as well as platform-specific attribution miss crucial aspects of consumer behavior and the true impact of media investments. Combine tools that reflect your full marketing mix.
Emergence of Advanced Tools. New tools such as Media Mix Modeling (MMM) and Incrementality experiments offer insights into long-term effects and test causality, adapting to current challenges.
Combining Strengths for Better Insights. A combined approach using various tools provides a more comprehensive view of media effectiveness and optimizes marketing budgets.
Innovative Solutions. Team up with measurment pioneers such as Kickbite to take advantage of innovations like the AI Click & View Attribution model, combining advanced statistic modeling with the benefits of datadriven attribution making it ideal for channel and tactic decision making.
It's fundamental to identify the analytics stage of your company. Does your team have the capabilities of interpreting the results of each tool? It is equally important to clearly define: What are the use cases of each tool before making any investment.
Decisions start with trust
14-days for free